VoltValet: 自律型モバイル EV 充電
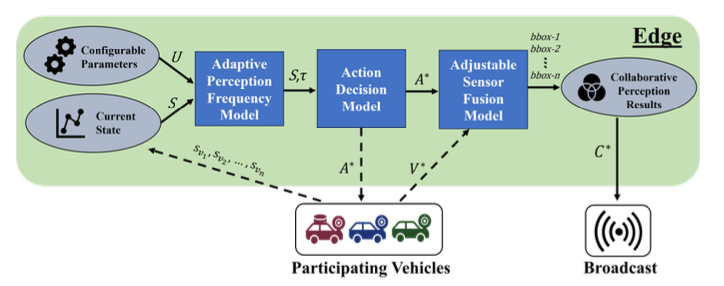
Background:
Modern vehicles come with varying levels of sensors and computing power. Some have advanced systems like LiDAR (a laser-based sensor for detecting objects in 3D) and onboard computers, while others rely solely on basic cameras or have no external perception at all. Collaborative perception allows multiple vehicles to share sensor data, enabling even older or less-equipped vehicles to benefit from a richer, more complete view of their surroundings. This improves road safety by detecting hazards earlier and enhances traffic efficiency by reducing blind spots and increasing situational awareness.
However, real-time collaborative perception is challenging due to network variability (different wireless speeds and reliability) and computing constraints (some vehicles can process data, others cannot). This research introduces HAdCoP (Heterogeneous Adaptive Collaborative Perception), a system that intelligently decides how and when vehicles should share sensor data. By dynamically managing data transmission, computing resources, and sensor integration, HAdCoP ensures fast, accurate, and efficient perception across a mixed fleet of vehicles.
Methods:
HAdCoP is designed with three key components that work together to optimize collaborative perception.
First, the CaLPeN (Context-aware Latency Prediction Network) decides which vehicles should send sensor data to prevent network congestion. It selects the most relevant perception tasks for each vehicle and determines whether processing should occur onboard (inside the vehicle) or be offloaded to an edge computing system (a roadside server with greater processing power).
Next, the APF (Adaptive Perception Frequency) Model adjusts how frequently vehicles update their perception based on real-time conditions such as network speed, traffic density, and available computing resources. This prevents unnecessary data transmission while maintaining perception accuracy and responsiveness.
Finally, the Sensor Fusion Model, based on the HEAL (Heterogeneous Alliance) framework, merges sensor data from different vehicles. Using machine learning, it intelligently combines information from LiDAR and cameras to create a comprehensive perception model that is more accurate than any single vehicle’s view.
To evaluate HAdCoP, the researchers combined vehicular perception data from the OPV2V dataset with real-world 5G network data, simulating two realistic traffic scenarios. The first, CSC1 (Current Era), assumes only a few vehicles have advanced sensors and computing. The second, CSC2 (Future Era), represents a world where most vehicles are equipped with LiDAR and onboard processing.
Findings:
The study showed that CaLPeN improved perception accuracy by 5.5% compared to other models, making collaborative perception significantly more effective. The APF model successfully adjusted perception update rates based on real-time conditions, ensuring reliable data sharing while preventing delays. By dynamically selecting which vehicles should participate, HAdCoP optimized accuracy while minimizing network load.
One of the key advantages of the system was its ability to balance edge computing and onboard processing. Vehicles with computing power processed data locally, while those without offloaded tasks to nearby edge servers, reducing latency and ensuring real-time operation.
Overall, these results confirm that collaborative perception can greatly enhance road safety by providing all vehicles with a shared, more accurate view of their environment.
Future Work:
Moving forward, researchers aim to refine data fusion techniques to further improve perception accuracy, especially in challenging conditions such as poor weather or heavy traffic. Additional security measures will be developed to protect the system from potential cyber threats. Finally, real-world testing with actual vehicles will help validate and fine-tune the framework, bringing it closer to deployment in next-generation intelligent transportation systems.
By enabling vehicles to work together as a networked intelligence system, this research moves us closer to a future of safer, smarter roads where every car—new or old—benefits from the latest perception technology.
Learn more at: https://ieeexplore.ieee.org/document/10852339
接続状態を維持
ミディアムとLinkedInで私たちの旅をフォローしてください。