VoltValet: 自律型モバイル EV 充電
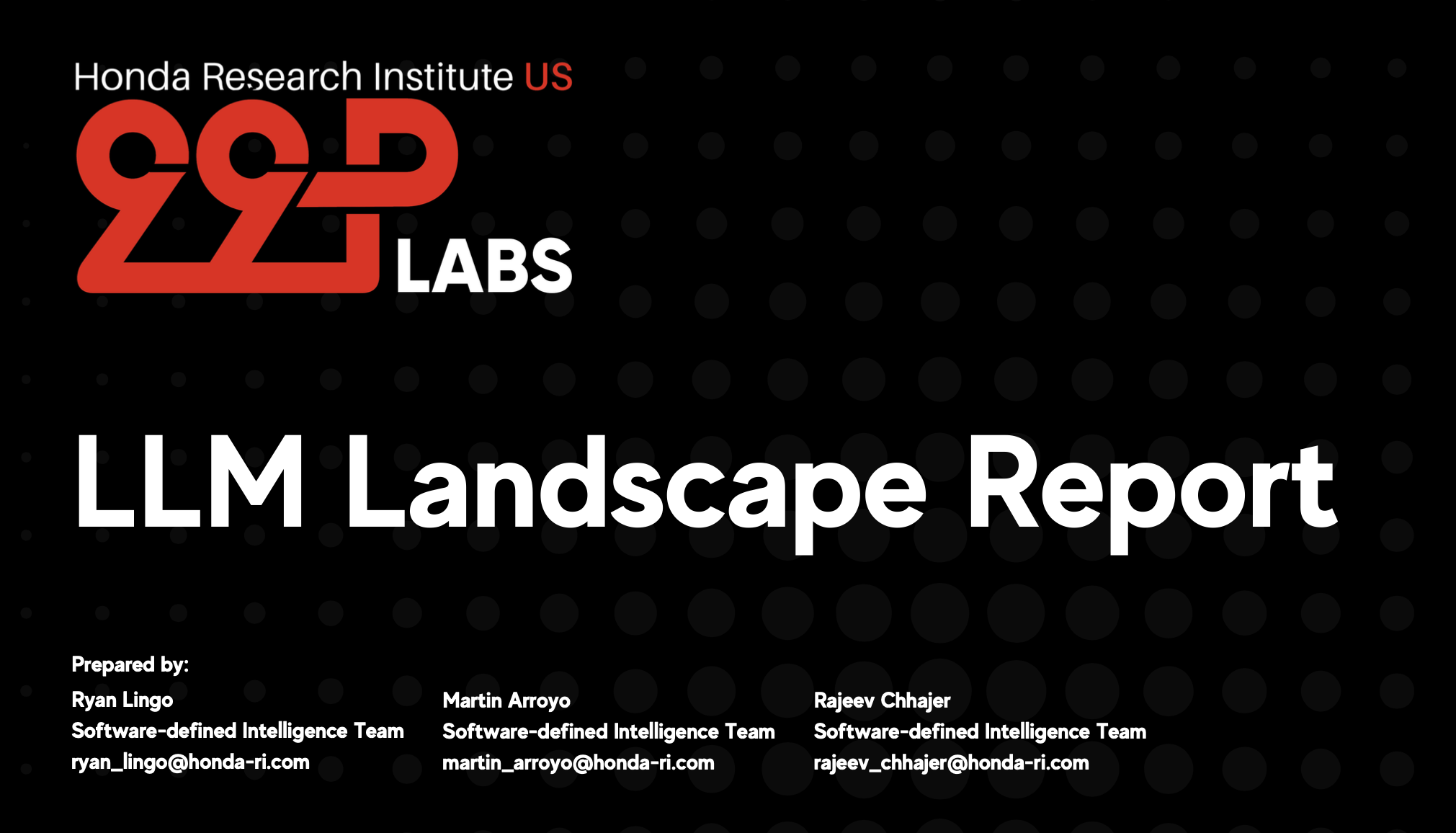
Background:
The LLM Landscape Report by 99P Labs provides an in-depth analysis of large language models (LLMs), highlighting industry trends, cutting-edge research, and practical applications. The report examines the growing role of LLMs in business, the impact of Retrieval-Augmented Generation (RAG), small model experimentation, and best practices for optimizing LLM performance. Additionally, it explores 99P Labs’ contributions to AI innovation, including structured problem-solving techniques and academic collaborations that drive real-world applications.
Methods:
The report synthesizes insights from ongoing research, industry developments, and university-led capstone projects. It covers key methodologies such as REAP (Reflection, Explicit Problem Deconstruction, and Advanced Prompting) to improve LLM reasoning, RAG implementations for enhanced retrieval accuracy, and fine-tuning strategies for domain-specific applications. Additionally, small model experimentation was conducted to assess the feasibility of running LLMs in resource-constrained environments, evaluating factors like cost, efficiency, and scalability.
Findings:
The findings demonstrate that LLMs are becoming core business tools, with techniques like RAG and fine-tuning significantly improving accuracy and contextual relevance. REAP-based structured reasoning methods led to measurable improvements in LLM problem-solving capabilities, while small model experiments showed that optimized, lower-cost models can perform competitively in targeted use cases. The report also highlights that hybrid approaches—combining retrieval systems, structured workflows, and prompt engineering—lead to more reliable and interpretable AI outputs, supporting broader adoption in industry and academia.
Download the report: Landscape Report
接続状態を維持
ミディアムとLinkedInで私たちの旅をフォローしてください。